Welcome to the International Institute of Tropical Agriculture Research Repository
What would you like to view today?
Development of a multi-layer gene regulatory network perturbation simulation model for host-pathogen interaction studies
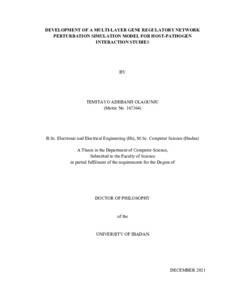
View/ Open
Date
2021-12Author
Olagunju, T.A.
Type
Review Status
Internal ReviewTarget Audience
Scientists
Metadata
Show full item recordAbstract/Description
Gene Regulatory Networks (GRNs) modulate the traits of an organism. Perturbation experiments which were employed to identify Trait-influencing Genes (TGs) are limited to only a few genes at once, and inadequate to identify the TGs of complex traits like disease resistance. Network modelling techniques for complex systems such as GRNs can provide a holistic system view to overcome the limitation of identifying TGs of complex traits with perturbation experiments when applied to genome-wide Next Generation Sequencing (NGS) data. This study was therefore designed to mine and identify disease-responsive genes from GRN using network perturbation technique. Small Ribonucleic Acid (sRNA) Profiles (sRNAP) from computationally annotated NGS data were combined with Gene Co-expression (GC) data and used to construct two-layer GRN Models (GRNMs). Node removal perturbation was applied to the GRNMs, and the Percentage Network Density Change (PNDC) was recorded as the network robustness measure and perturbation response. Model validation was done in three stages. The sRNAP was compared with two Published Profiles (PP) using number of Conserved sRNAs (CsRNAs), Cleaned Raw Sequences (CRS), Host sRNAs (HsRNAs) and Pathogen-derived sRNAs (PsRNAs) as parameters. The GRNMs were validated using F-Score and p-value from Analysis of Variance (ANOVA). Well-defined Gene Ontology (GO) annotation was used for biological interpretation of the results. The model was applied to GC data containing 3,146 genes, and NGS data comprising 383,105,237 sequences from five Cassava genotypes labelled as A, B, C, D and E. An automated computational pipeline was developed to annotate the NGS data across all the dataset and produced the sRNAP comprising 25,214 sRNAs and 16,436 genes involved in 105,515 interactions, used for constructing the two-layer differential GRNMs. The PNDC for ten differential GRNMs AB, AC, AD, AE, BC, BD, BE, CD, CE and DE were, -0.0086, 0.3140, -0.1315, -0.2204, - 0.1519, 0.0649, -1.6422, -0.0895, -0.6397 and -0.3999, respectively, indicating AB as the most robust and BE the least to node removal perturbation. The CsRNAs for sRNAP was 144 contrasted with 114 and 118 in the two PPs, while the CRS for sRNAP was 97.09 compared to 87.46 and 65.70 % in PP. The HsRNAs in the sRNAP ranged from 71.14 to 89.00, but were 65.90 to 73.51 and 66.90 to 70.69 % in PP. The PsRNAs range was 9.87 to 23.56, while 4.00 to 17.00 and 7.34 to 12.65 % were reported in the two PPs. The F-Score for the randomly rewired GRNMs was between 4.49 at 0.03 pvalue and 1934.00 at <2e-16 p-value, while it was between 5.26 at 0.02 p-value and 728.9 at <2e-16 pvalue for the randomly relabelled GRNMs, suggesting that the GRNMs were truly representative of the underlying biological network. The GO annotation revealed that the perturbed nodes which resulted in reduced network robustness were disease-responsive genes. The developed perturbation simulation model identified disease-responsive genes obtained through the reduced network robustness measures validated by gene ontology. This knowledge could be useful in reprogramming the gene regulatory network to obtain desirable traits.
Acknowledgements
I acknowledge my supervisors Dr Angela U. Makolo and Dr A. Gisel, first for believing in me, and then for the patience in guiding me through the journey of this research work. Painstakingly, I have absorbed like a sponge all the mentoring and
capacity-building efforts and I remain eternally grateful for these. Furthermore, I acknowledge the International Institute of Tropical Agriculture (IITA) Graduate Research Fellowship awarded to me by Dr A. Gisel to execute this research work.
I would also ...