Welcome to the International Institute of Tropical Agriculture Research Repository
What would you like to view today?
Integrating spatial heterogeneity to enhance spatial temporal crop yield predictions
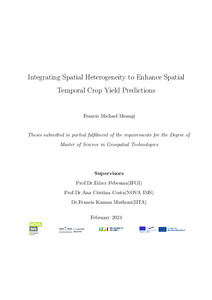
View/ Open
Date
2024-02Author
Msangi, F.M.
Type
Review Status
Internal ReviewTarget Audience
Scientists
Metadata
Show full item recordAbstract/Description
Crop yield predictions and monitoring are important in understanding key challenges
in crop production and management to ensure the effective utilization of resources to
enhance food security. Over the years remote sensing data and machine learning models
have been employed with the help of ground truth data as reference in the estimation of
crop yields across space and time. However, the common machine learning methods often
overlook the spatial heterogeneity inherent in regions leading to sub-optimal estimations.
Moreover, the transferability of the machine-learning model to new environments is rarely
addressed during spatial-temporal predictions.
This study integrates spatial heterogeneity by utilizing the Geographically weighted random
forest model(GWRF). It investigates whether accounting for heterogeneity can improve
spatial-temporal predictions of crop yields and estimate the area of applicability of
the models. The models are tested with maize yield data from farms practising conservation
agriculture(CA) and another group applying the farmers’ conventional practices(CP)
in Zambia and Malawi. The GWRF is compared to the ordinary Random Forest(RF)
model using environmental blocking cross-validation.
The overall performance of the GWRF was better compared to the standard RF model
with RMSE of 1587.731 kg/ha and 1389.206 kg/ha for the CA and CP respectively. The
coefficient of determination (R2) was 0.171 and 0.234 for CA and CP respectively.
Acknowledgements
I would like to express my special thanks of gratitude to Almighty God for granting me
knowledge and good health throughout.
I would also like to appreciate the support offered by my supervisors and Co-supervisors;
Prof.Dr Edzer Pebesma, Prof. Dr. Ana Cristina Costa and Dr Francis Kamau Muthoni
for their time and guidance from the beginning to the end of my research. Sincere thanks
should also go to the International Institute of Tropical Agriculture (IITA) and CIMMYT
for their great support ...