Welcome to the International Institute of Tropical Agriculture Research Repository
What would you like to view today?
Evaluating the dry matter content of raw yams using hyperspectral imaging spectroscopy and machine learning
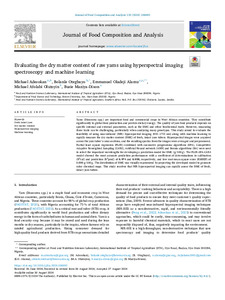
View/ Open
Date
2024-11Author
Adesokan, M.
Otegbayo, B.
Alamu, E.O.
Olutoyin, M.A.
Maziya-Dixon, B.
Type
Review Status
Peer ReviewTarget Audience
Scientists
Metadata
Show full item recordAbstract/Description
Yams (Dioscorea spp.) are important food and commercial crops in West African countries. They contribute significantly to global food production and provide dietary energy. The quality of yam food products depends on specific internal and external parameters, such as the DMC and other biochemical traits. However, measuring these traits can be challenging, particularly when analyzing many genotypes. This study aimed to evaluate the feasibility of using near-infrared (NIR) hyperspectral imaging (932–1721 nm) along with machine learning to rapidly measure the dry matter content (DMC) of fresh, intact yam tubers. Hyperspectral images were acquired across the yam tuber’s cross-sections, and the resulting spectra from the images were averaged and preprocessed. Partial least square regression (PLSR) combined with successive progressions algorithms (SPA), Competitive Adaptive Reweighted Sampling (CARS), Artificial Neural network (ANN) and Boruta algorithms (BA) were used to select the important wavelengths for developing a prediction model for DMC (g/100 g). The PLSR-SPA-CARS model showed the most accurate prediction performances with a coefficient of determinations in calibration (R2cal) and prediction (R2pred) of 0.974 and 0.958, respectively, and low root mean square error (RMSEP) of 0.898 g/100 g. The distribution of DMC was visually represented by projecting the developed model to generate color chemical maps. This study resolves that NIR hyperspectral imaging can rapidly assess the DMC of fresh, intact yam tubers.
https://doi.org/10.1016/j.jfca.2024.106692
Multi standard citation
Permanent link to this item
https://hdl.handle.net/20.500.12478/8543IITA Authors ORCID
Michael Adesokanhttps://orcid.org/0000-0002-1361-6408
ALAMU Emmanuel Oladejihttps://orcid.org/0000-0001-6263-1359
Busie Maziya-Dixonhttps://orcid.org/0000-0003-2014-2201
Digital Object Identifier (DOI)
https://doi.org/10.1016/j.jfca.2024.106692